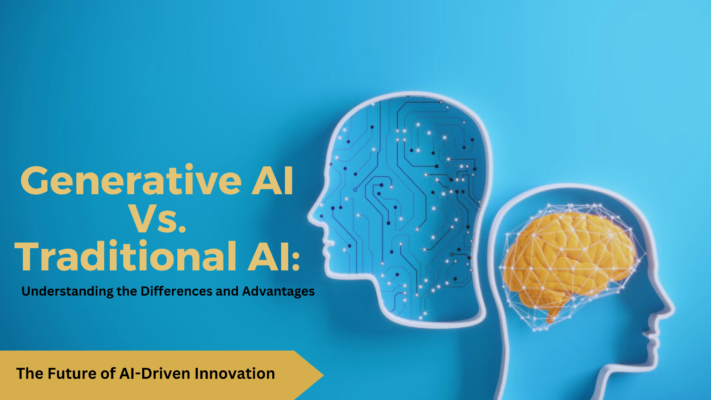
- April 11 2024
- admin
Introduction: The Changing Landscape of AI
In the ever-evolving world of artificial intelligence (AI), the landscape has witnessed a remarkable transformation in recent years. Enterprises have long relied on traditional AI approaches, which have played a crucial role in driving innovation and unlocking new opportunities. However, the emergence of Generative AI has ushered in a new era, redefining the boundaries of what is possible with AI-driven technologies.
As enterprises navigate this dynamic landscape, the distinction between Generative AI and Traditional AI has become increasingly important. In this comprehensive blog post, we will explore the key differences, advantages, and challenges associated with these two distinct approaches to AI, empowering enterprises to make informed decisions and capitalize on the immense potential of these transformative technologies.
Understanding Generative AI: The Future of AI-Driven Innovation
Generative AI, a revolutionary subset of artificial intelligence, has captured the attention of enterprises worldwide. This innovative approach to AI goes beyond the traditional capabilities of pattern recognition and data analysis, empowering machines to generate original content, engage in open-ended dialogue, and solve complex problems in ways that were previously unimaginable.
At the core of Generative AI lies the ability to learn from vast datasets and generate new, high-quality outputs that are tailored to specific contexts and user needs. This is achieved through the use of advanced deep learning models, such as Generative Adversarial Networks (GANs) and Variational Autoencoders (VAEs), which enable machines to create content that is both coherent and creative.
The Rise of Generative AI
The rapid advancements in Generative AI can be attributed to several key factors, including:
- Availability of Large Datasets: The exponential growth of data, driven by the digital revolution, has provided Generative AI models with the necessary fuel to learn and create.
- Increased Computing Power: The availability of powerful GPU-accelerated computing resources has enabled the training and deployment of increasingly complex Generative AI models.
- Breakthroughs in Deep Learning: Innovations in deep learning architectures, such as Transformer models and Diffusion models, have significantly improved the quality and versatility of Generative AI outputs.
- Democratization of AI Tools: The emergence of user-friendly Generative AI tools and platforms has lowered the barriers to entry, empowering a wider range of enterprises to leverage these transformative technologies.
Generative AI in Action
Generative AI has already demonstrated its potential across a wide range of applications, revolutionizing how enterprises approach various tasks and challenges. Some of the most prominent use cases of Generative AI include:
- Content Generation: Enterprises are leveraging Generative AI to create high-quality and personalized content, such as articles, marketing materials, and even computer-generated imagery and videos.
- Conversational AI: Generative AI-powered chatbots and virtual assistants are transforming the way enterprises interact with customers, providing more natural and contextual responses.
- Predictive Analytics: Generative AI models are being used to generate synthetic data, which can be used to train more accurate predictive models and simulate complex scenarios.
- Process Automation: Enterprises are harnessing Generative AI to automate repetitive tasks, such as document summarization, code generation, and task completion.
- Creative Problem-Solving: Generative AI is empowering enterprises to tackle complex challenges in innovative ways, from product design and prototyping to scientific research and drug discovery.
Traditional AI: The Foundations of Enterprise Innovation
While the rise of Generative AI has captured the spotlight, traditional AI approaches have been the bedrock of enterprise innovation for decades. These well-established techniques, rooted in fields like machine learning, rule-based systems, and expert systems, have been instrumental in driving transformation across various industries.
The Strength of Traditional AI
Traditional AI approaches have a proven track record of delivering tangible business value, with a focus on solving specific, well-defined problems. Some of the key strengths of Traditional AI include:
- Reliability and Robustness: Traditional AI models are often built on a solid foundation of statistical analysis and domain-specific knowledge, making them more reliable and stable in production environments.
- Interpretability and Explainability: Traditional AI techniques, such as decision trees and linear regression, tend to be more transparent and easier to interpret, enabling enterprises to understand and trust the decision-making process.
- Regulatory Compliance: Many industries, such as finance and healthcare, have stringent regulatory requirements, and Traditional AI approaches are often better equipped to meet these standards.
- Specialized Domain Knowledge: Traditional AI solutions are often tailored to specific industries or use cases, leveraging deep domain expertise to deliver highly accurate and targeted results.
The Application of Traditional AI
Traditional AI has been instrumental in driving innovation and transformation across a wide range of enterprise domains, including:
- Predictive Analytics: Traditional machine learning models, such as regression and classification algorithms, have been widely used for forecasting, risk assessment, and customer segmentation.
- Automation and Optimization: Rule-based systems and expert systems have been deployed to automate repetitive tasks, optimize workflows, and improve operational efficiency.
- Decision Support: Traditional AI-powered decision support systems have been employed to assist human experts in making informed and data-driven decisions.
- Computer Vision and Image Processing: Traditional computer vision techniques, such as edge detection and object recognition, have been extensively used in various industries, from manufacturing to healthcare.
- Natural Language Processing: Rule-based NLP approaches, including pattern matching and language models, have been instrumental in powering enterprise applications like chatbots and text analysis.
The Convergence of Generative AI and Traditional AI
While Generative AI and Traditional AI may appear to be distinct and opposing approaches, the reality is that they are increasingly converging, with enterprises leveraging the strengths of both to drive innovation and achieve their business objectives.
Complementary Capabilities
Generative AI and Traditional AI can be viewed as complementary technologies, each offering unique capabilities that can be harnessed to address various enterprise challenges.
Generative AI excels in tasks that require creativity, adaptability, and open-ended problem-solving, such as content generation, conversational AI, and predictive analytics. Traditional AI, on the other hand, shines in areas that demand reliability, interpretability, and specialized domain knowledge, such as automation, decision support, and computer vision.
By embracing the convergence of these two approaches, enterprises can unlock a powerful synergy, leveraging the strengths of both to create innovative solutions that are tailored to their specific needs.
Hybrid Approaches
Enterprises are increasingly adopting hybrid approaches that seamlessly integrate Generative AI and Traditional AI, creating a powerful combination that can deliver unparalleled results.
One such example is the integration of Generative AI models, such as language models, with Traditional AI techniques, like rule-based systems and knowledge bases. This hybrid approach enables enterprises to generate high-quality, contextual content while ensuring that the output adheres to specific business rules and regulatory requirements.
Another example is the use of Generative AI for data augmentation, where synthetic data generated by Generative AI models is used to train and improve the performance of Traditional AI models, particularly in scenarios with limited real-world data.
By embracing hybrid approaches, enterprises can leverage the best of both worlds, harnessing the creativity and adaptability of Generative AI while maintaining the reliability and interpretability of Traditional AI.
The Evolving Landscape
As Generative AI and Traditional AI continue to converge, the landscape of enterprise AI is undergoing a transformative shift. Enterprises are recognizing the importance of striking the right balance between the innovative capabilities of Generative AI and the proven strengths of Traditional AI.
This convergence is driving the emergence of new AI architectures, tools, and frameworks that seamlessly integrate these two approaches, empowering enterprises to tackle a wider range of challenges and unlock new avenues for growth and competitive advantage.
Key Advantages of Generative AI vs. Traditional AI
As enterprises navigate the evolving landscape of AI, understanding the key advantages of Generative AI and Traditional AI is crucial for informed decision-making and strategic planning. Let’s explore the distinct benefits of each approach:
Advantages of Generative AI
- Creativity and Innovation: Generative AI models excel at generating original and highly creative content, from engaging text and images to novel product designs and scientific discoveries. This enables enterprises to push the boundaries of what is possible and stay ahead of the competition.
- Adaptability and Versatility: Generative AI models are designed to be adaptable and versatile, capable of tackling a wide range of tasks and challenges across various domains. This agility allows enterprises to leverage Generative AI for a diverse set of use cases, from content creation to predictive analytics.
- Personalization and Customization: Generative AI models can be fine-tuned and customized to cater to the unique needs and preferences of individual users or specific enterprise contexts, enabling more personalized and tailored experiences.
- Rapid Prototyping and Experimentation: The ability of Generative AI to quickly generate new ideas, content, and solutions empowers enterprises to rapidly prototype and experiment, accelerating the innovation process and reducing time-to-market.
- Scalability and Efficiency: Generative AI models can be deployed and scaled efficiently, leveraging the power of modern computing resources to generate high volumes of content or insights in a timely and cost-effective manner.
Advantages of Traditional AI
- Reliability and Robustness: Traditional AI models are often more reliable and robust, with a proven track record of delivering consistent and stable results in production environments.
- Interpretability and Explainability: Traditional AI techniques tend to be more transparent and easier to understand, allowing enterprises to gain insights into the decision-making process and build trust in AI-powered solutions.
- Regulatory Compliance: Many industries have strict regulatory requirements, and Traditional AI approaches are often better equipped to meet these standards, ensuring that enterprises can operate within the confines of relevant laws and guidelines.
- Specialized Domain Knowledge: Traditional AI solutions are frequently tailored to specific industries or use cases, leveraging deep domain expertise to deliver highly accurate and targeted results.
- Operational Efficiency: Traditional AI-powered automation and optimization solutions have been instrumental in driving operational efficiency and cost savings for enterprises across various sectors.
Balancing Innovation and Legacy: Navigating the Synergies
As enterprises seek to harness the power of AI to drive innovation and maintain a competitive edge, the challenge lies in striking the right balance between the transformative potential of Generative AI and the proven capabilities of Traditional AI.
Integrating Generative AI and Traditional AI
Enterprises should strive to create a harmonious integration between Generative AI and Traditional AI, leveraging the unique strengths of each approach to address their specific business needs.
This integration can take various forms, such as:
- Hybrid AI Architectures: Enterprises can develop AI solutions that seamlessly combine Generative AI and Traditional AI models, enabling the complementary capabilities of both to be harnessed within a single system.
- Augmented Intelligence: Enterprises can employ Generative AI to enhance and augment the capabilities of Traditional AI-powered systems, such as using Generative AI to generate personalized content or enhance the decision-making process.
- Iterative Development: Enterprises can adopt an iterative approach, starting with Traditional AI solutions to address well-defined problems and then gradually incorporating Generative AI capabilities to drive innovation and tackle more complex, open-ended challenges.
By carefully integrating Generative AI and Traditional AI, enterprises can create a synergistic ecosystem that capitalizes on the strengths of both approaches, unlocking new levels of efficiency, creativity, and competitive advantage.
Navigating the Challenges
As enterprises embrace the convergence of Generative AI and Traditional AI, they will also need to navigate a range of challenges, including:
- Talent and Skill Development: Enterprises must invest in upskilling their workforce to ensure that they have the necessary expertise to effectively leverage and manage both Generative AI and Traditional AI technologies.
- Governance and Ethical Considerations: Enterprises must establish robust governance frameworks and ethical guidelines to ensure the responsible and transparent deployment of AI technologies, addressing concerns around bias, transparency, and data privacy.
- Integration and Compatibility: Enterprises must overcome the technical challenges of seamlessly integrating Generative AI and Traditional AI solutions, ensuring smooth data flow, interoperability, and scalability.
- Regulatory Compliance: Enterprises must navigate the evolving regulatory landscape, ensuring that their AI-powered solutions adhere to industry-specific regulations and standards, particularly in highly regulated sectors.
- Security and Resilience: Enterprises must prioritize the security and resilience of their AI systems, protecting against potential vulnerabilities and ensuring the reliability and integrity of their AI-powered applications
By proactively addressing these challenges and fostering a culture of continuous learning and experimentation, enterprises can successfully navigate the convergence of Generative AI and Traditional AI, positioning themselves for long-term success and sustainable innovation.
The Future of Enterprise AI: Embracing the Convergence
As we look toward the future, the convergence of Generative AI and Traditional AI is poised to play a pivotal role in shaping the landscape of enterprise AI. Enterprises that embrace this convergence and strategically leverage the unique strengths of both approaches will be well-positioned to drive innovation, maintain a competitive edge, and adapt to the rapidly evolving business landscape.
Driving Transformative Innovation
The synergistic integration of Generative AI and Traditional AI will enable enterprises to drive transformative innovation across a wide range of industries. Generative AI will empower enterprises to push the boundaries of creativity and open-ended problem-solving, while Traditional AI will provide the reliability, interpretability, and specialized domain knowledge necessary to ensure the practical application and scalability of these innovations.
Enhancing Operational Efficiency
By leveraging the convergence of Generative AI and Traditional AI, enterprises will be able to enhance their operational efficiency through intelligent automation, optimization, and decision support. Generative AI can be used to generate personalized content, streamline processes, and assist human experts in making data-driven decisions, while Traditional AI can ensure the reliability and compliance of these AI-powered solutions.
Fostering Adaptive and Resilient AI Ecosystems
As the enterprise AI landscape continues to evolve, the convergence of Generative AI and Traditional AI will enable the development of adaptive and resilient AI ecosystems. These ecosystems will seamlessly integrate the creative and versatile capabilities of Generative AI with the robust and specialized knowledge of Traditional AI, empowering enterprises to adapt to changing market conditions, regulatory environments, and customer demands.
Delivering Personalized and Contextual Experiences
The convergence of Generative AI and Traditional AI will pave the way for enterprises to deliver highly personalized and contextual experiences to their customers and stakeholders. Generative AI will enable the creation of tailored content, products, and services, while Traditional AI will ensure that these experiences are aligned with the specific needs and preferences of the target audience.
Accelerating the Pace of Enterprise Innovation
By embracing the convergence of Generative AI and Traditional AI, enterprises will be able to accelerate the pace of innovation, rapidly prototyping and iterating on new ideas, products, and business models. This agility and adaptability will be crucial in an increasingly dynamic and competitive business landscape, allowing enterprises to stay ahead of the curve and capitalize on emerging market opportunities.
Conclusion: Embracing the Convergence for Sustained Success
In the ever-evolving world of enterprise AI, the convergence of Generative AI and Traditional AI represents a transformative opportunity for organizations seeking to drive innovation, maintain a competitive edge, and adapt to the rapidly changing business landscape.
By understanding the unique strengths and capabilities of both Generative AI and Traditional AI, enterprises can strategically leverage the synergies between these two approaches, creating a powerful and versatile AI ecosystem that can address a wide range of challenges and unlock new avenues for growth.
As enterprises navigate this convergence, it is crucial to strike a careful balance, integrating the innovative potential of Generative AI with the reliability and specialized knowledge of Traditional AI. This harmonious integration will enable enterprises to deliver transformative solutions, enhance operational efficiency, foster adaptive and resilient AI ecosystems, and provide personalized and contextual experiences to their customers and stakeholders.
By embracing the convergence of Generative AI and Traditional AI, enterprises can accelerate the pace of innovation, remain agile in the face of change, and solidify their position as leaders in their respective industries. The future of enterprise AI lies in the seamless convergence of these two powerful approaches, empowering organizations to achieve sustained success and drive innovation that transcends the boundaries of the status quo.